
Mark Germaine
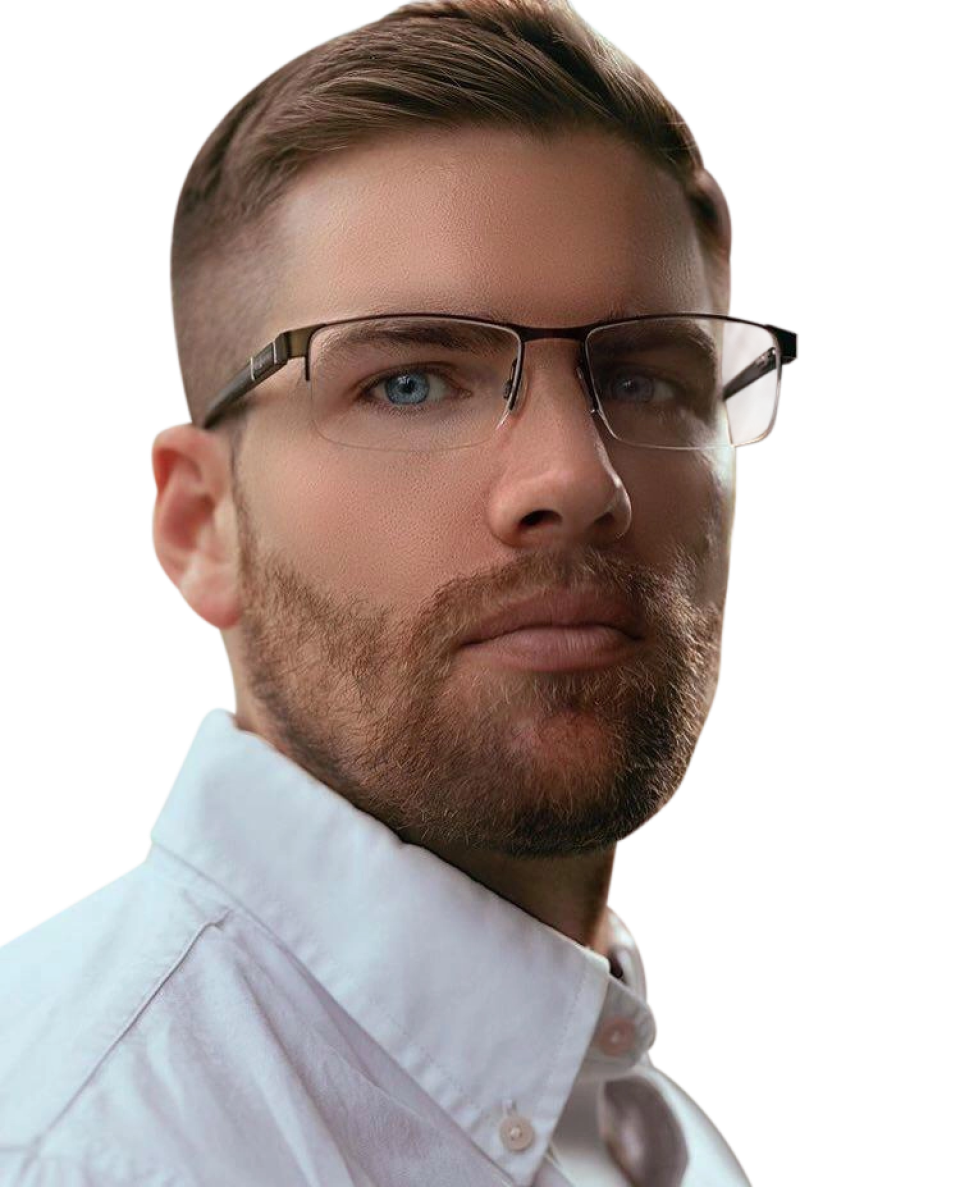
Mark Germaine
Name: Mark Germaine
Contact Number: No office number
Email address: mark.germaine2@mail.dcu.ie
Room Number: L2 (INSIGHT)
Qualifications to date: BSc. (hons) Sport Science and Health (TUD), MSc. Sport Nutrition (LJMU)
Research Title: Early detection of gestational diabetes risk using explainable machine learning models
Year Commenced: 2021
Anticipated year of completion: 2025
Supervisor: Dr. Brendan Egan & Dr. Graham Healy
Research Summary:
Gestational diabetes mellitus (GDM) is defined as glucose intolerance of varying severity and is present in ~12% of Irish pregnancies (O’Sullivan et al. 2011). Women with GDM are at increased risk of adverse health and delivery outcomes leading to complications, including stillbirth, premature delivery, macrosomia, fetal hyperinsulinemia, and clinical neonatal hypoglycemia (Dunne et al. 2012, Danyliv et al. 2015, Gillespie et al. 2013). Consequently, maternity care costs for pregnant women who develop GDM are 34% higher than the average pregnancy (Gillespie et al. 2013), whereas neonatal costs in the first year of life are typically higher than those in offspring of mothers without GDM (Chen et al. 2009).GDM is most commonly diagnosed during weeks 24-28 of the pregnancy and is diagnosed via an oral glucose tolerance test (OGTT) which is a simple test that measures blood glucose response over a period of 2 hours in response to the ingestion of 75g of glucose (ADA). Effective intervention in GDM has beneficial effects for maternal and neonatal outcomes (Damm et al. 2016, Plows et al. 2018), thus if machine learning models can accurately predict the risk of GDM at an earlier date, this may allow for earlier intervention from clinicians, thus potentially reducing health risks to both mother and child, reducing the risk of complications during pregnancy and saving money in the treatment of GDM. Some studies have tried to assess the risk of GDM by measuring fasting blood glucose during the first trimester, however the results have not been promising (Zhu et al. 2012). Further to this, researchers have been attempting to predict the risk of GDM from much earlier in pregnancy, from 8 to 20 weeks in Chinese women (Zheng et al. 2019) using simple models such Bayesian logistic regression. More recently, researchers have applied simple and advanced machine learning models on variables extracted from medical records in China in an attempt to predict GDM (Wu et al. 2021). Whilst some of these models have very high specificity, sensitivity varies between the models applied and the best sensitivity achieved was 70% using a deep neural network. In Ireland, routine care appointments are scheduled between weeks 8-12, thus the variables for the model will be collected during this period as this is when standard care data is collected and thus more data available. The ideal scenario being that the model would have a strong predictive value by week 12 of the pregnancy, half of the time usually taken to detect GDM. The aim of the current research project would be to explore the applicability of machine learning models for predicting the risk of GDM in pregnant women in Ireland. The objectives of this research are to:1) explore the utility and applicability of machine learning models on predicting the risk of GDM based on variables collected during routine pregnancy care.2) assess the efficacy of the machine learning in practice if the results show high accuracy.3) try to achieve this using explainable machine learning
Acknowledgements: Science Foundation Ireland Research Centre for Training in Machine Learning