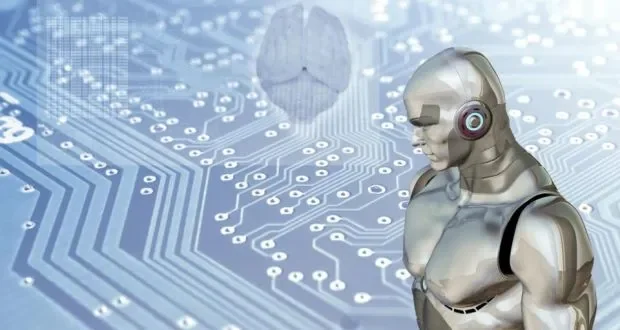
Artificial intelligence is dead: long live data analytics
Artificial intelligence fires the human imagination. Tales of man-made intelligence are peppered throughout literature and cinema history, from Mary Shelley’s Frankenstein to the earliest science fiction movies. People are fascinated by the idea of creating intelligence, of engineering the very essence of what it is to be human.
So is it here? Are we living in the age of AI? Many would argue that we are.
There are lots of examples of computer technologies showing incredible leaps in progress in recent times after decades of research and development.
They do exhibit some intelligence. Think about language translation software. We speak English to a phone app, and the app translates what is said into French, Spanish or any of dozens of languages, including Mongolian.
Software translates speech into text when you speak to a phone, or a watch, without training on a particular voice, and it even works in environments with noisy backgrounds such as in a restaurant or outdoors on a windy day.
Image recognition
Automatic recognition of image and video content is now much more than just recognising faces in pictures: it now assigns captions or tags to describe what is in the picture. Facebook uses this to make images accessible to the blind and Google Photos use it to tag personal photos.
IBM’s Watson system can read in text documents and answer questions about their content. Watson was fed the entire contents of Wikipedia and competed in the US game show Jeopardy against two previous champions. Watson won.
Jeopardy is like a cross between University Challenge and Only Connect, requiring extensive real world knowledge, and clever analysis of language. Watson is now being applied by IBM to medicine and scientific literature to help users understand the huge volume of scientific information being produced daily.
Think also of self-driving cars, soon to be navigating our roads, avoiding obstacles, including each other, in order to take us safely and economically to our destinations.
These examples, and many others, are all being touted as forms of AI.
But are they AI? Well, no actually. Companies like calling their technologies AI. It sounds better, it’s more futuristic, but it’s not AI: it’s actually data analytics.
IBM’s Watson, for example, achieves what it does by analysing sentences to draw connections across sentences, paragraphs, documents. What makes it clever is that it does this for really complex text, and it does it at enormous scale, processing vast amounts of data.
It is not reading and understanding in the way we envisaged an AI machine would.
Nowadays, we have computers performing tasks that require the equivalent of human intelligence. Back in the day it was thought that this would require a certain type of processing: a deep semantic representation of meaning and complex inference.
It turns out that sheer brute force data analytics cuts the mustard just as well. The other examples of AI given earlier in this piece also take a data driven approach. We just never realised how far we could get by going down that road.
It’s a shame for AI. The field has long been crippled by the cycle of inflated expectations and disappointing results.
Stop-start research
In 1967 Marvin Minsky, co-founder of MIT’s AI laboratory, proclaimed that the problem of AI would be substantially solved within a generation. Minsky’s optimism proved to be misplaced and by the 1970s, agencies funding this research had become frustrated at the lack of progress. As a result, funding all but dried up.
The field recovered in the early 1980s, but again fell victim to massive expectations and by 1987 nobody wanted to fund AI research. The AI winter closed in yet again. Research stalled.
Yet in the interim, computers got faster, with more data storage capacity and started crunching numbers, bigger numbers of numbers, and crunching them faster.
We now know that pattern and correlation recognition, data mining and machine learning actually create capabilities we thought would require human intelligence, to complete tasks such as autonomous driving and so on.
Data analytics, not AI, is the most important field of computing today. Yet, we need to learn from our past and not fall into the trap of creating hype by making inflated claims about what is no more than just fast computers reading patterns from huge data volumes.
Then again, the end product is the same. AI researchers were working towards computers that could learn, that could understand language, that could solve problems. Those things exist now. Maybe we are in the age of AI after all. It just took data analytics to get us here.
As Bones McCoy from Star Trek might have said, “It’s AI Jim, but not as we know it.”
Alan Smeaton is professor of computing and director of the Insight Centre for Data Analytics at Dublin City University.
Irish Times 29th July 2016.